Genomics Coming of Age: Accelerating A Cure Part 2 of 2
Monday August 17, 2020By Julie DiBene
Director, Marketing Communications
MoSys, Inc.
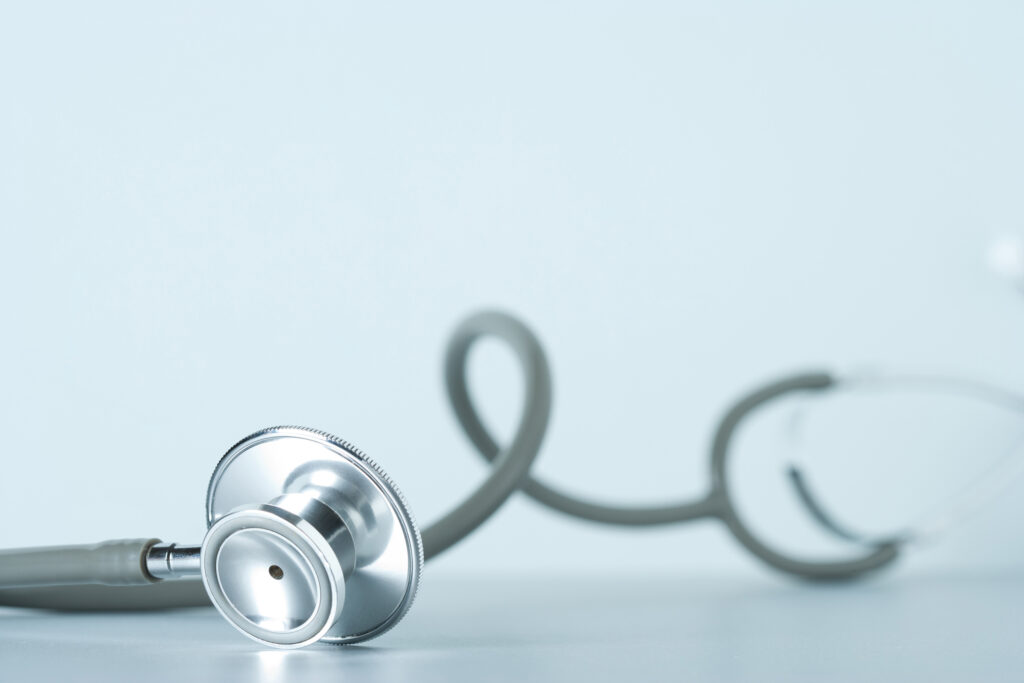
In Part I of this blog, we covered some of the major advances that genomics has made in the study of genes associated with the cure and prevention of diseases. In Part 2, we will cover how MoSys Graph Memory Engine (GME) technology can accelerate genomics and ultimately, a cure.
In a study, published this past April in the Journal of Virology, researchers used computer models to predict which combination of HLAs genes might be best at binding SARS-CoV-2, and which might be worst. It’s a milestone for sure however there is still much more research to be done because the human body is far more complex than what is being discussed here. Medical experts would like to use the immune response data already culled to compare with genetic data gathered from the same patients. They seek to pair COVID-19 testing with HLA typing, to try and predict how different HLA types will react to COVID symptoms, if at all. Aligning the data with genetic testing companies, biobanks and organ transplant registries could also offer chances to study HLA types in larger populations of people but time is running out for so many people already infected. Time is what so many don’t have so running these massive calculations, processing and assessing enormous amounts of data as rapidly as possible is key.
Now back to the MoSys solution. We have a Graph Memory Engine (GME) IP for walking down graph representation of problem sets. The GME can be implemented in software, on standalone FPGAs and FPGAs accelerated with the MoSys Programmable HyperSpeed Engine IC. The PHE utilizes a proprietary high random-access rate memory with in-memory-compute for searching and classifying in support of applications such as genomics, bioinformatics, and network security. The PHE is a monolithic IC, a Programmable Hyper-Speed Engine (PHE), with 1 Gb of internal memory, a bandwidth of ~1.5 Tb/s, to support a system with superior execution requirements.
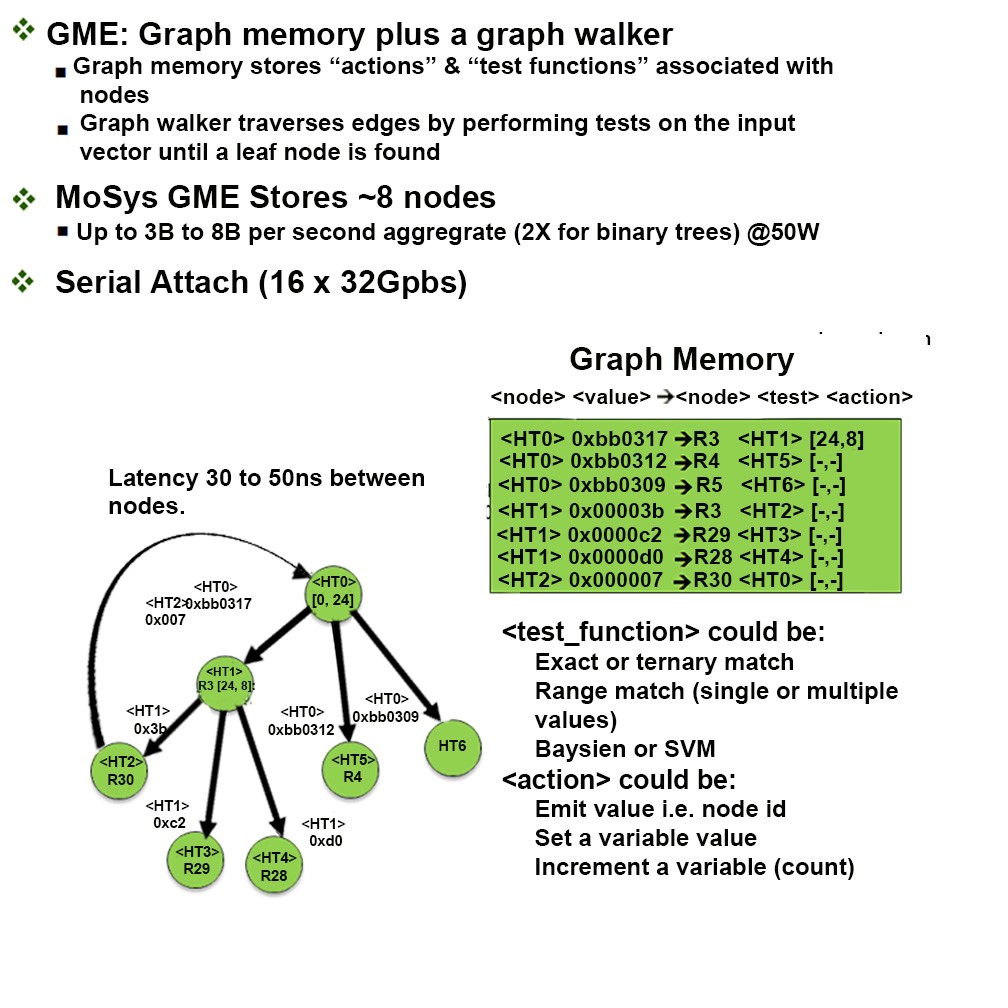
The result is a graph or tree that can be processed at blazing fast 3 to 6 Billion nodes per second with an average latency of ~30-50 ns per node in a multi-threaded environment. Performance in computing algorithms used in hierarchical data structures, like random forest of trees, decision trees, and graphs, is dependent upon the number and depth of the nodes/trees required to perform a classification and the memory access rate. Processing these algorithms is memory-bound and not compute-bound because each decision in the simplest form is a numeric comparison. The performance of GPUs and CPUs will improve as memory access rates improve. Since the rates of DRAM have marginally improved while the volume of data, processor speed, and algorithm efficiency have increased significantly, external memory solutions will not fix this bottleneck, unless memory acceleration is implemented. It is now vital that the medical community be able to address these data bottlenecks and move forward with their research as quickly as possible. Acceleration technology has become a tool that researchers can leverage to find, sort, retrieve, process and analyze data faster than ever before. People’s very lives may now depend on it.
If you are looking for more technical information or need to discuss your technical challenges with an expert, we are happy to help. Email us and we will arrange to have one of our technical specialists speak with you. You can also sign up for updates. Finally, please follow us on social media so we can keep in touch.
Additional Resources:
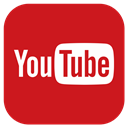
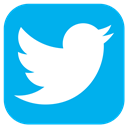
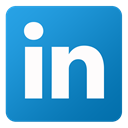